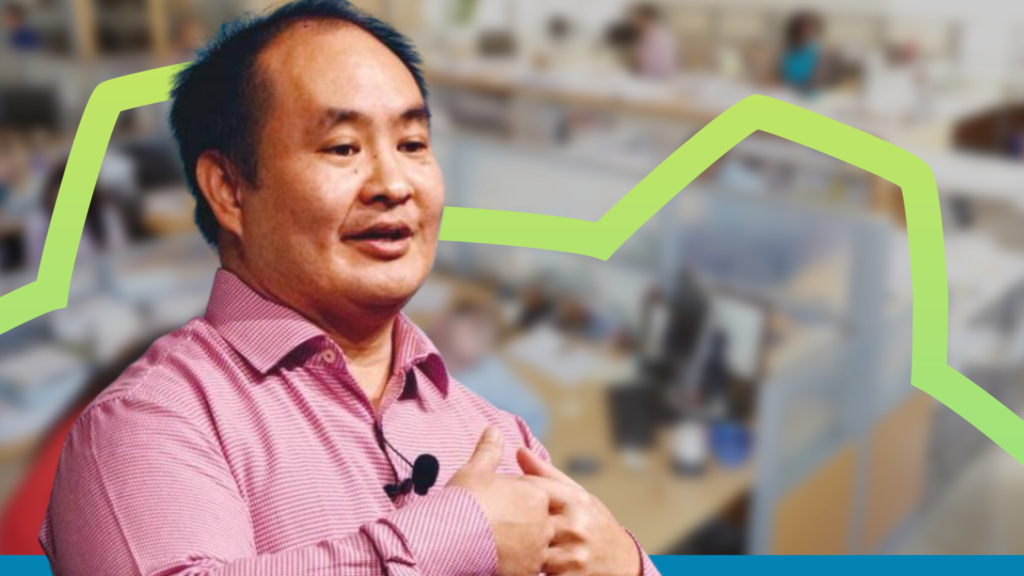
There’s so much data, from so many different sources, with so many different reporting tools, that you could just drown in reports, attribution, and meetings. With so much noise out there, it’s important that you look at the data in a certain way. There’s important information hidden in the metrics that will help direct your digital marketing strategy.
In this article I’m going to walk you through this technique that I’ve been using for 25 years, called MAA.
Metrics, Analysis, Action
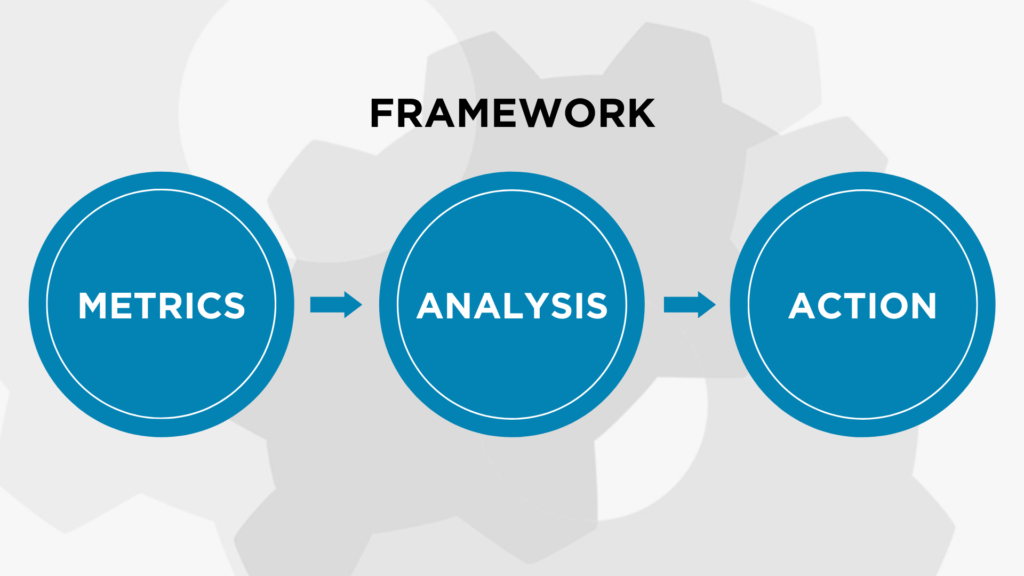
MAA stands for metrics, analysis, action.
Let me show you how powerful it is when you use this technique on any kind of data set you have. It could be SEO data, website data, email data, conversion data, shopping cart data.
The Data Doc is in…
Think of this as if you are a surgeon in the emergency room. You must follow these three steps.
- Collect vitals.
- Diagnose.
- Treat.
First you collect the vitals. It could be heart rate, blood pressure, respiratory rate, x-rays things like that. These are the numbers that clue you in to the cause of the problem.
The second phase is the diagnosis. In this phase you interpret all the vitals that you collected. Based on the data, you make the determination of a heart attack, broken bone, virus, etc. The key point is that the diagnosis is based on the data.
From that diagnosis, you create the treatment plan. The plan might include surgery, medications, a recovery plan, etc. But the list of things to be done to make the patient healthier is based upon the findings and the diagnosis.
The marketing analytics data you collect leads directly to analysis of the problem. That then leads directly to the action. What I will show you in this article is a number of examples from a variety of digital marketing projects. This works whether you’re working on a large or small project.
Data vs Analytics
Lots of people think that they have analytics because they have Google Analytics installed on their website.
But let me tell you a dirty secret.
There are no analytics in Google Analytics. It’s just Google charts. It should be called Google Chart-Maker.
Marketing analytics is figuring out what’s actually going on. It’s the interpretation of the data. Interpreting the data tells you why sales went up or down. It helps you discover why conversion rates went up or down. Analyzing the data answers questions like:
- Why did people buy or not buy?
- Why did a competitor take a certain action?
- Where are we losing customers along the customer journey?
- Is our content hitting or missing with our customers?
Analytics is more than making charts and collecting data. And action is the next step after marketing analytics.
The way we see it, if you are not taking action based on the analytics, which was based on the data, then whatever you’re doing is random.
Returning to our analogy, not everyone should take the same pill. If you’ve got a broken bone, you shouldn’t take the same medication as someone who has a headache. So the action that you take, the optimization, should be contingent upon the analysis, which should go straight back to the data that you gathered.
Most people make the mistake of just trying to look at lots of data. This Metrics Analysis Action framework is the easiest way to figure out what you really need to do versus what’s noisy.
MAA Framework Case Study: Ecommerce
If you are in ecommerce, lead gen, or any kind of performance marketing, then you’re going to start with the action, mapped back to the analysis, and back to the metrics.
Because the actions are all the things that you could do.
So make a list of the things that you could do.
- You can play with the website.
- You can change your budgets.
- You can change ads.
- You can optimize creatives.
- You can work with influencers.
- You can buy another tool.
- You can change bids.
Think of all the actions that you could take. Start with the end in mind.
Once you decide on the action, look for the trigger. In other words, when analyzing the data, what diagnosis will cause you to take that prescribed action?
That’s where you have automated rules on Google, Facebook, or Shopify. Wherever you’re looking at data, you can set up these rules.
For example, if your cost per acquisition goes above $50, then turn the ad set off. If someone leaves a positive review on Yelp, then reach out to them to say thank you.
So if a certain thing happens, then here’s the particular action.
Then there’s a limited number of things that you could do, so you don’t have to look at everything. And then if you need to determine if that triggering condition is true, then what data do you need?
Data, Analytics, and Attribution
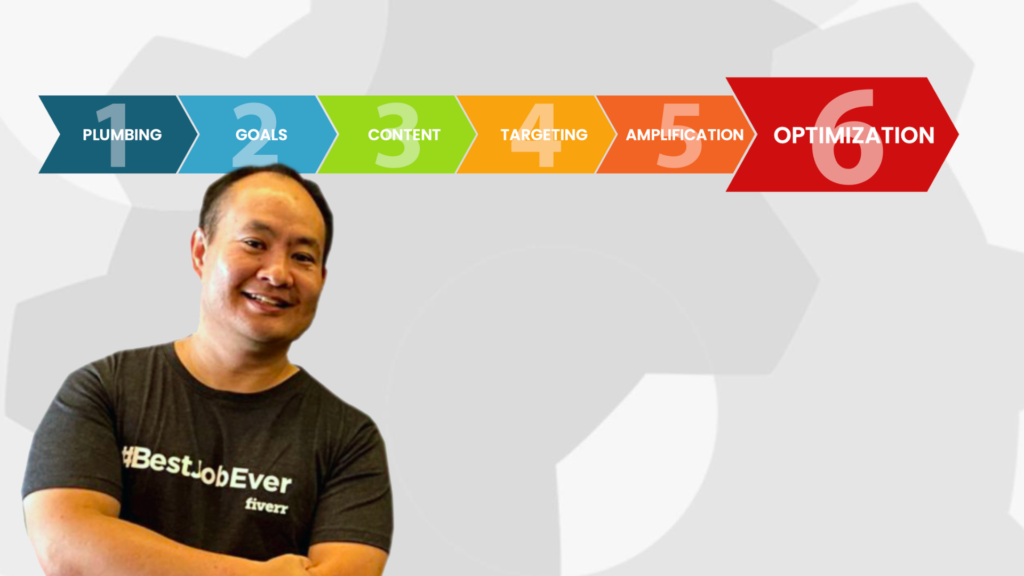
On the far left of this image, we have plumbing. Plumbing is collecting the data from different tags in tag manager, UTM parameters, pixels that are firing, and other events inside an app.
These are the things that people are doing. For example, opening an email. When that happens, you get plenty of email marketing data. But the data doesn’t mean anything unless you can tie it to a goal.
How do you tie data to a goal?
Here’s a lifetime value example…
Seeds of Life sells flowers to people who’ve experienced the death of a loved one. The lifetime value (LTV) of a customer is $150. What can they do to increase the LTV?
They might offer a referral bonus, free shipping for orders over a hundred dollars, etc. Their goals, checked against the marketing analytics, will determine the direction of their next marketing campaign.
The important thing is to define the goals and measure them against the data. If the data doesn’t tie to the achievement of a particular goal, then you have to ask, “why are we even collecting that data?”
We’re not searching for a needle in a haystack, here. Although, that’s what most people do with their reporting.
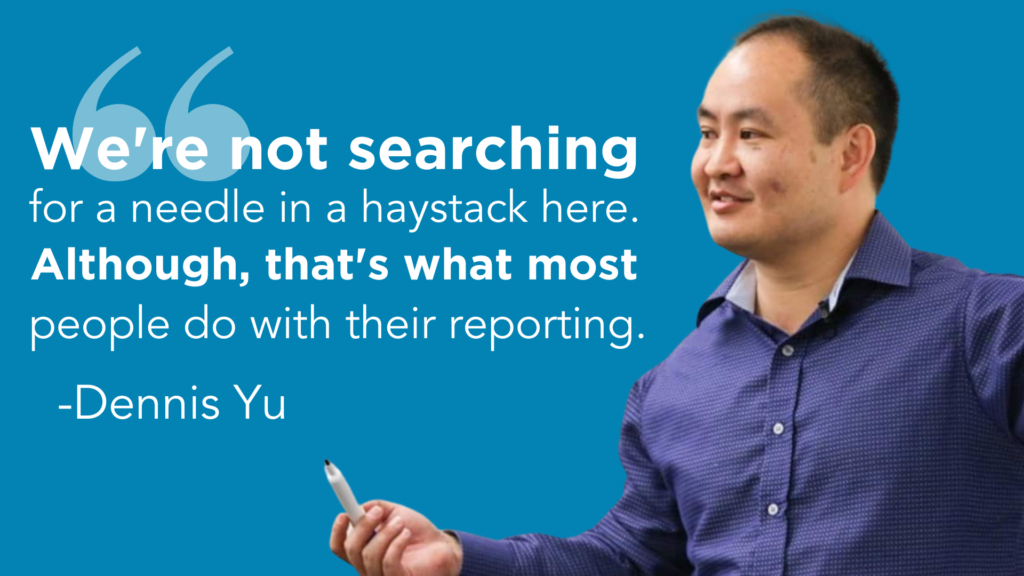
Most people log into Google analytics, or whatever they use to pull in all the data from all the different places. And then they just hunt and peck and wander around and look for interesting things.
They look at the data then filter down to this date for that particular segment and this part of the country. It’s like the lotto, like the power ball where you choose six random balls to try to win the million dollar jackpot.
You want to have your goals before you figure out the plumbing.
Don’t Make the Same Mistakes with Analytics
Large and small companies make the same mistakes. They tend to go after impressions or click through rate or secondary metrics when the primary metric, the business goal, is more important than a diagnostic, secondary metric.
I love looking at cost per mille, or CPM, in advertising. For example, how much are you paying per thousand impressions? What is the trigger or check engine light, to let you know whether the algorithm is penalizing you for having a low click through rate, low quality score, low relevance score, etc.
Analyzing a marketing campaign in this way may show that something else is wrong.
Please don’t make the same mistake thinking that a secondary metric like click through rate, cost per click, quality score, or CPM is more important than the main business metric.
Profit, lifetime value, or cost of acquisition should be the goals that tie to your content and targeting.
Plumbing, Goals, Content, Targeting, Amplification, Optimization…
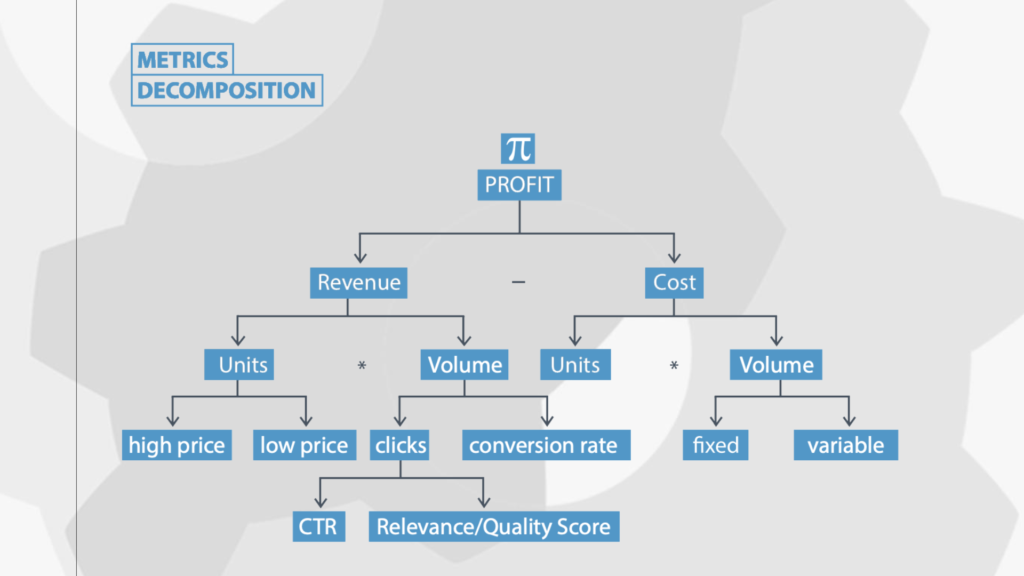
Here’s an example (above) of a marketing campaign we ran for our friend, Brennan.
At the very top are the financial metrics, specifically profit. There’s some kind of margin with or without cost of goods and services or overhead.
Then we have revenue minus costs.
Revenue is driven by factors like conversion rate, LTV, and how well you use things like recency and frequency to increase revenue.
Then there’s costs: people costs, ad costs, software costs, other kinds of costs.
On the revenue side, units (high price vs low price) multiplied by volume (clicks and/or conversion rate) is your revenue.
On the cost side, let’s say you run all your digital marketing campaigns on a cost per click basis. You can break that down to different fixed and variable costs. So we know if we double the number of clicks we’re buying from Google, we’re going to pay twice as much. Multiply the cost by the number of clicks you get for the overall cost of that campaign.
This decomposition pyramid helps you figure out the data you need to collect using secondary diagnostic metrics.
Start to think about how those different metrics will help you uncover the main issue to focus on right now.
MAA Framework: Case Study
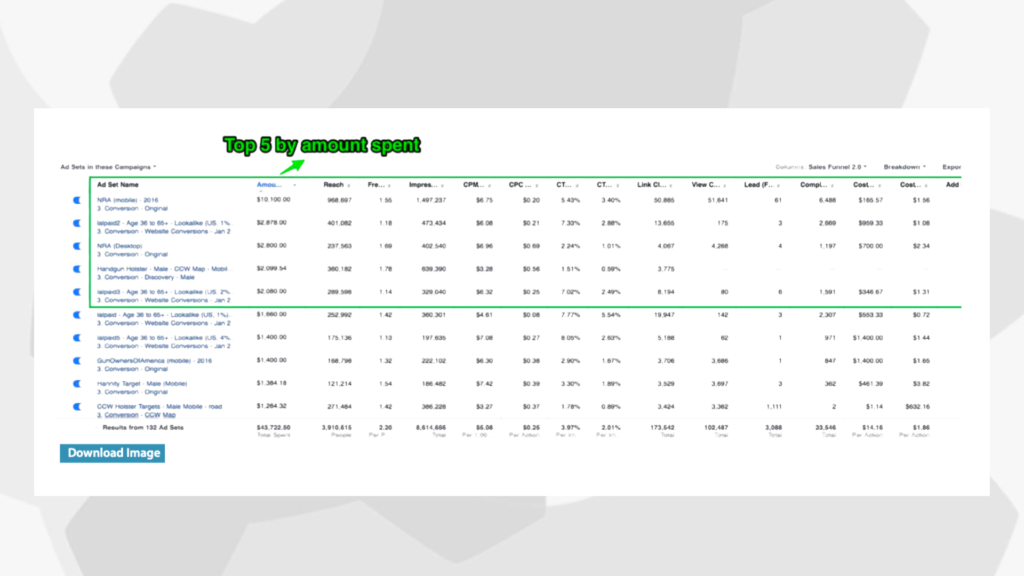
Let’s look at how this actually applies when you’re looking at tabular data.
In this example (above), we’re looking at a lot of information. There are 132 ad sets here. That means we have all this information for 132 projects…
- Data
- Campaigns
- Ads
- Landing pages
- Messages
This happens to be a set of Facebook campaigns, but it could easily be any social media platform or other traffic source.
We use a concept called “Top N” to select a manageable number of ad sets to work with. Why? Because it’s intimidating to try and look at ALL of them to diagnose the problem or issue.
You don’t have time to look at every single keyword, creative, or landing page. The idea of Top N is to look at the top, best- or worst-performing ad sets and ignore the rest. This is just another way of using the 80/20 rule or prioritizing your work.
I find that when you use the Top N technique on any large dataset you can quickly zero in on the most important thing.
In this case, we can see that this very first ad spent $10,000 out of $43,000. That means 25% of all of the money being spent is inside that one ad out of the 132 ads total.
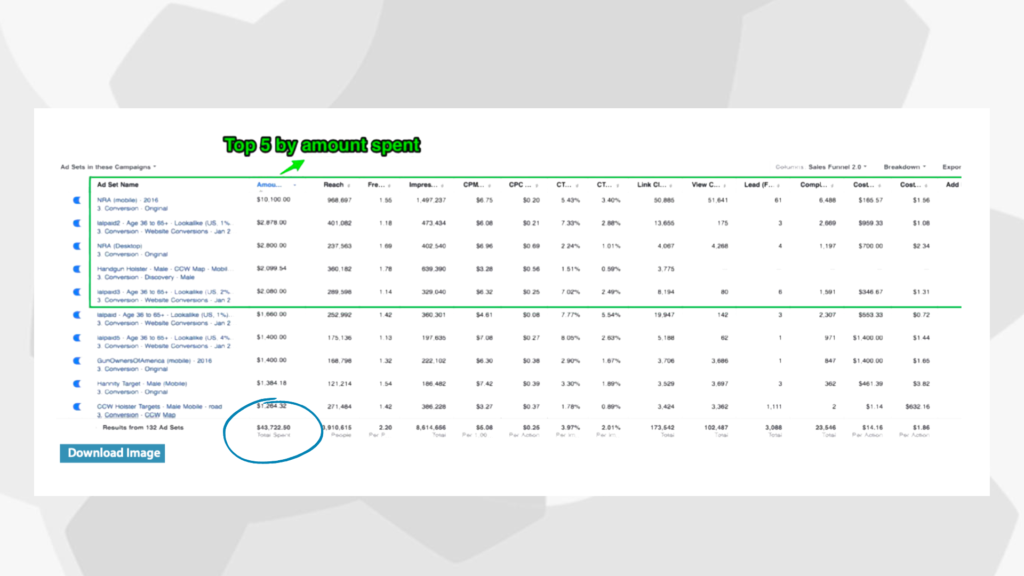
Look a little more closely and you’ll see the top five already account for 60% of the total spend.
That’s not uncommon. In lots of cases the top three to five ads will account for about half of your ad spend.
Applying the Top N Method
I like to start by doing Top N on spend, because that’s where I can identify a “bleeder” (a high-spend ad with very low return).
Then I look at what drove the most revenue or had the highest number of conversions. Because then I can find where the winners are.
Then I look at clicks, leads, or other metrics that are important to the business.
Using this method, I kill the losing ads and amplify the winning ads.
Let’s say you were to sort just by conversions or revenue. If you do that, then you could have an ad that’s wasting lots of money that doesn’t make it into the top four or five for your most important metrics.
So I use Top N for three or four metrics in succession. Each time it reorders the ad sets or ads or creatives or whatever it is that you’re looking at.
You can use this method to determine ad performance in just three minutes.
Find and Fix the Issue
If something’s out of whack, it could require a big change or it could be something wrong with the tracking.
It could be iOS 14, or the pixel wasn’t on that landing page. It could be the data didn’t come through and it’s delayed. There’s all kinds of things that could play into why numbers aren’t adding up.
A lot of people freak out when sales are way down. Understandable. But many times it’s because of some silly issue. So before you pull the fire alarm, just think, does that really make sense?
I like this particular ad here.
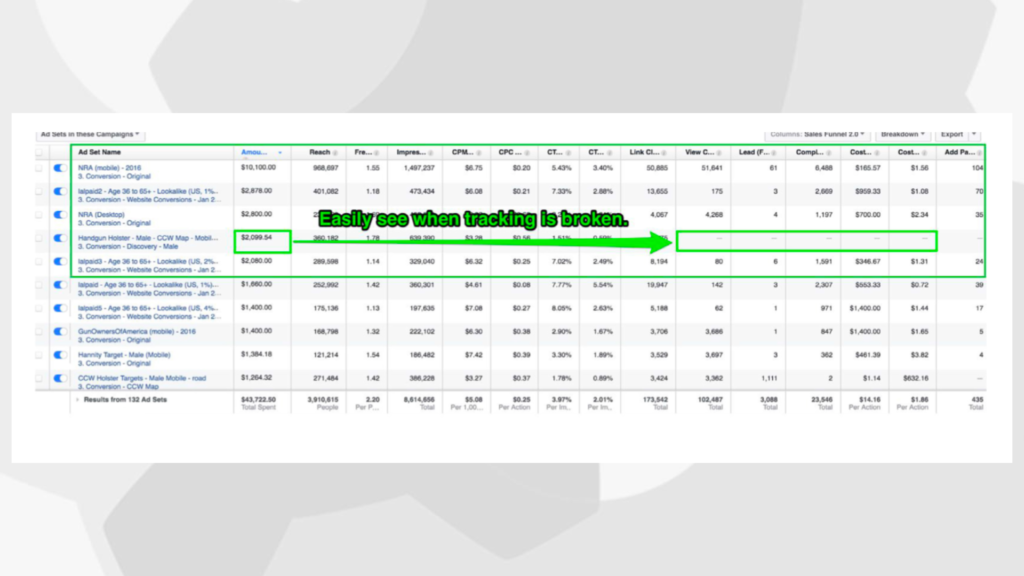
There’s no way we spent this amount of money with no return. So we know there’s an issue. And we know with social media platforms like TikTok, Twitter, and Facebook, their systems often will not show data.
We know that because of the iOS 14 update, impressions and clicks are reported on different frequencies. So you might see a bunch of spend show up before the conversions show up or vice versa.
Make sure it’s statistically significant. Also make sure that you have enough data, so you don’t jump to any conclusions.
We’ve seen these systems spiral out of control. For example, let’s say you decide to reduce the bid amount on a marketing channel when the ROI falls below a certain amount. That seems logical. But if you’re only looking at revenue, not conversions, you might kill off a marketing campaign that was actually working quite well.
Imagine if it all boiled down to a hiccup in the data that caused the downward spiral. Not good. So be careful about that.
Now, if you see that a metric is out of whack and the data looks good, then ask yourself why that campaign isn’t performing as well.
Data and Instinct for the Win
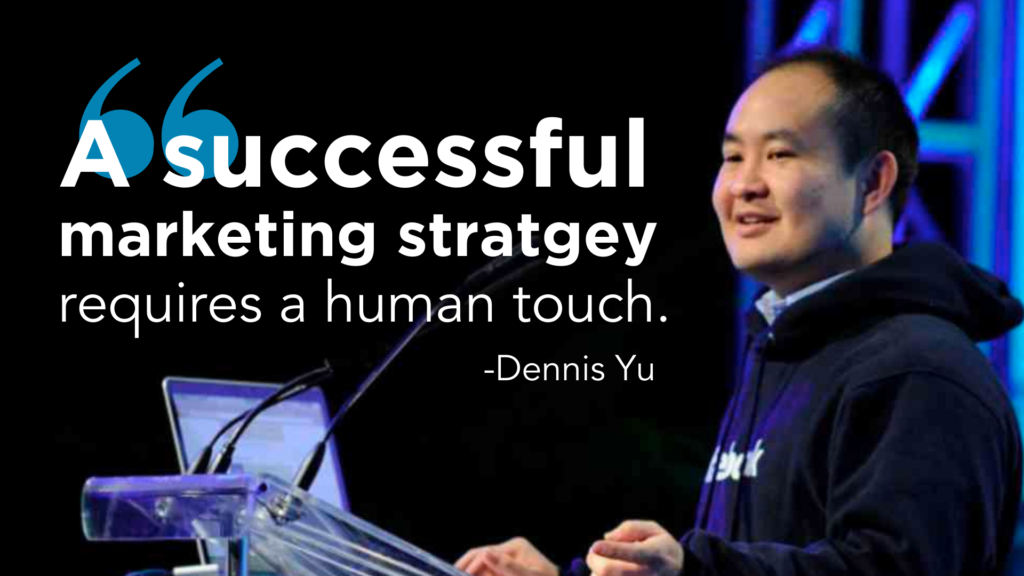
Don’t let everything you do be completely automated and dependent upon rules. A successful marketing strategy requires a human touch.
Don’t set so many rules that the software automatically terminates your ads.
Instead, take a moment to look at how far out of bounds the ad performance is. It could be that you launched a new campaign and you’re doing an AB test or some kind of split test. The winner stays on and continues to win, even when other ads are losing, because you’re trying to find another winner to take its place.
If the cost per acquisition is high, then you can break that down using the metrics decomposition pyramid.
For example, the cost per acquisition will double if:
- the conversion rate is cut in half and the cost per click is the same
- the cost per click doubles and the conversion rate is the same
The cost per acquisition remains the same if either factor doubles while the other one is cut in half.
Always look at your marketing analytics when the cost per conversion goes up. Determine whether it’s because of the cost per click or the conversion rate.
When you run ads using objective-based bidding you don’t have to worry as much about cost per click, click through rate, or conversion rate because the artificial intelligence behind the ad platform is going to seek your target metric.
If the target metric is out of whack, you can decompose it into the underlying metrics.
That’s true for organic traffic. But it’s not as true for paid traffic because the systems are getting smarter and can optimize for the objective you set. Either way you should still look.
Balancing Metrics
This method gets you to look at metrics that matter according to our business goals. It gets you to think about and analyze why the data might be good or bad. And it gets you to outline the actions you’re going to take when goals aren’t being met. Over time you’ll find that the same pairing of metrics change alongside each other. So let’s talk about what these balancing metrics are.
One company we were working with was spending a hundred thousand dollars a month on advertising. When they were unhappy with the return, the analyst on the project adjusted the Google ad campaign. All of a sudden the cost per conversion dropped from $20 per lead to $7 per lead.
But I wanted to know how and why it dropped so dramatically. I found out that this person went into the Google ads campaign and turned off all the campaigns except for the brand search terms. Of course it was going to convert super well!
But the balancing metric was volume. When the analyst “fixed” the cost per conversion, the number of leads dropped from 5,000 leads a month to maybe a thousand leads a month.
The key takeaway here is that if you optimize one metric blindly, you can fool yourself into thinking everything is better when in reality another metric took a nosedive.
Analyzing Like a Scientist, but NOT a Rocket Scientist
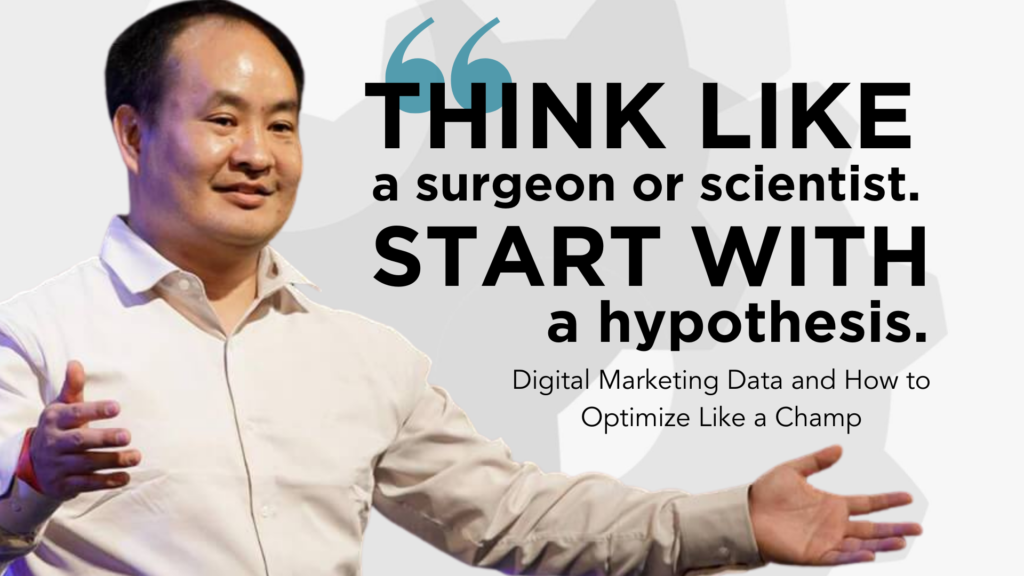
Metrics don’t matter, unless there’s a clear analysis that can come from the information. Remember, you’re seeking a diagnosis.
Think like a surgeon or scientist. Start with a hypothesis. If a certain thing happens, what will you do to correct it and what outcome do you expect? If there’s no potential action based on some metric, there’s no need to gather the metrics.
I see companies spend most of their efforts collecting data. No one even knows why they’re using the data. Be strategic and ask, “what are we doing with this data? Is there some meaningful action we’re going to take?”
Maybe there’s another metric that would measure the goal better.
The point of analytics is to figure out whether something is worthwhile. Most of the data you thought was important, doesn’t even matter.
I’ll give you one example. Our client was a large company, but this works for small companies, too.
We were working with an airline, taking one database and matching it against another. They wanted to know things like whether a customer that goes skiing has kids and what their income was.
They wanted predictive models to uncover which customers would be most likely to sign up for their credit card or buy flowers or upgrade or travel to new destinations.
We went all in on the idea that more data is better. After all the time and money spent on sophisticated data models, what we found was that the best predictor of people flying more was past purchase behavior. Not a surprise, right?
In this case, purchase behavior predicted purchase behavior. And the fact that they drove a station wagon, or liked to eat Haagen-Dazs ice cream, might be interesting but it had very little impact on their flying behavior.
Moral of the story, you might find that the most obvious thing is the best place to start optimizing in your business as well. Start thinking about what kind of “if-then” logic you can implement. And don’t dismiss the really simple idea just because it’s simple.
The MAA Framework is Not Just for Advertising
Collecting data allows you to put if-then sequences in place across your business. In Google and Facebook you can set up automated rules using if-then logic. For example, one might be for conversions. If conversions fall below a certain number, then an automated action would be taken or an alert might be sent to whoever’s in charge of that area to let them know there is something that needs their attention.
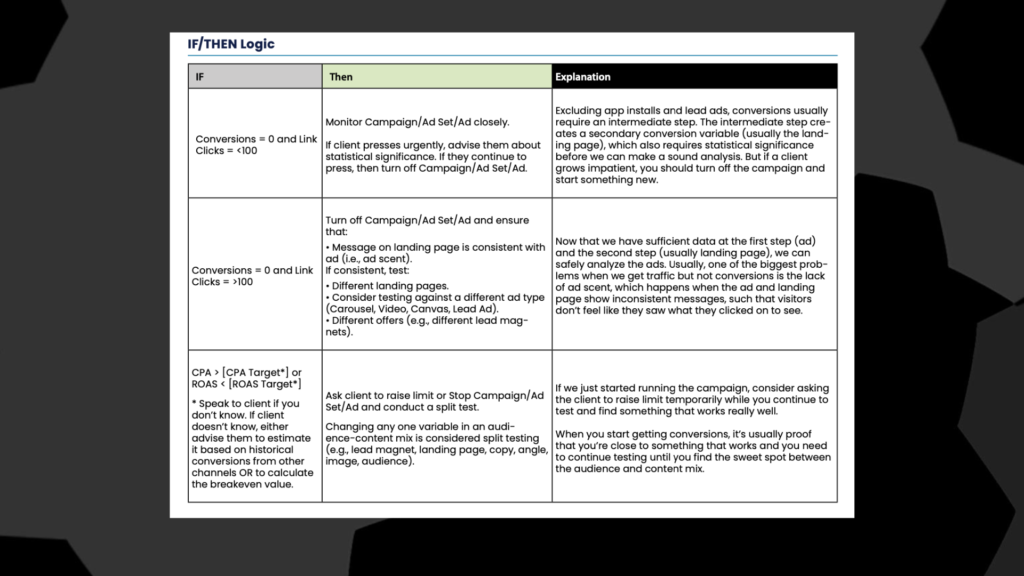
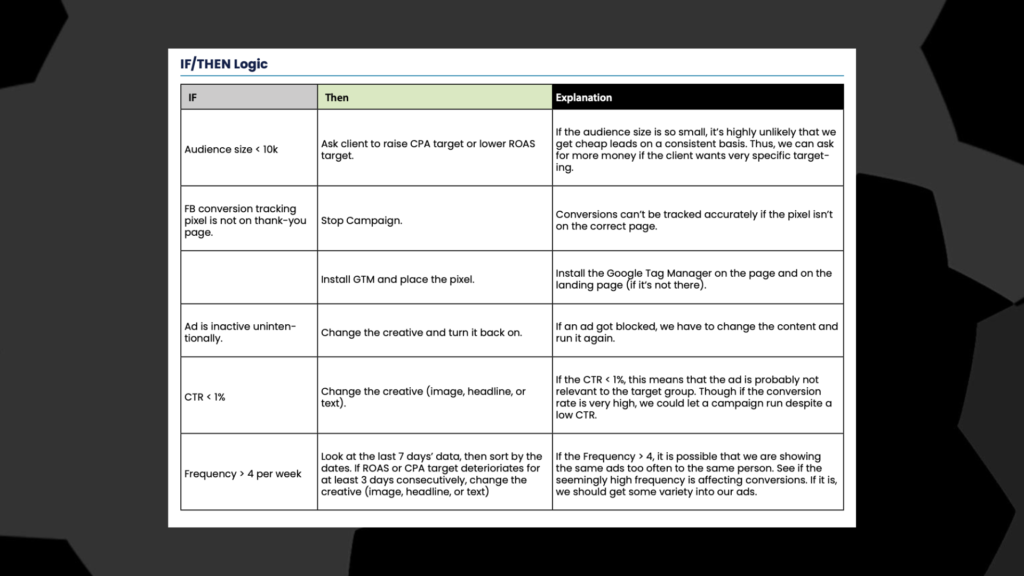
Here is a table of common if-then scenarios we’ve come across. Start small by looking at just a few of these things.
You’ll find a lot of value when you look at the patterns. For example, look at posts with the highest engagement versus posts with the lowest engagement. What can you learn? What do the high-engagement posts have in common? Is there a cross-over with the low-engagement posts?
Don’t spend all your time messing around inside the tools. Even Google’s head of analytics said that 90% of every dollar you spend on analytics should be on people and 10% should be on the tools.
We see a lot of businesses do the opposite. They spend 90% on tools and 10% on people. The hard truth is, the most sophisticated tools are useless without someone who knows how to make sense of the numbers.
To ensure success, set the framework in place. Make it clear that everyone is accountable for the results.
Summary
I hope the metrics, analysis, action framework I’ve just introduced you to encourages you. Data and analytics aren’t really that technical. You don’t have to collect a ton of data, build regression models, or feed your AI any recipes.
Customers buy this over that. It’s not math. It’s not huge databases. It’s not engineering.
The MAA framework is all about understanding the numbers in the context of business performance and goals. Tracking metrics should always begin with the business strategy in mind.
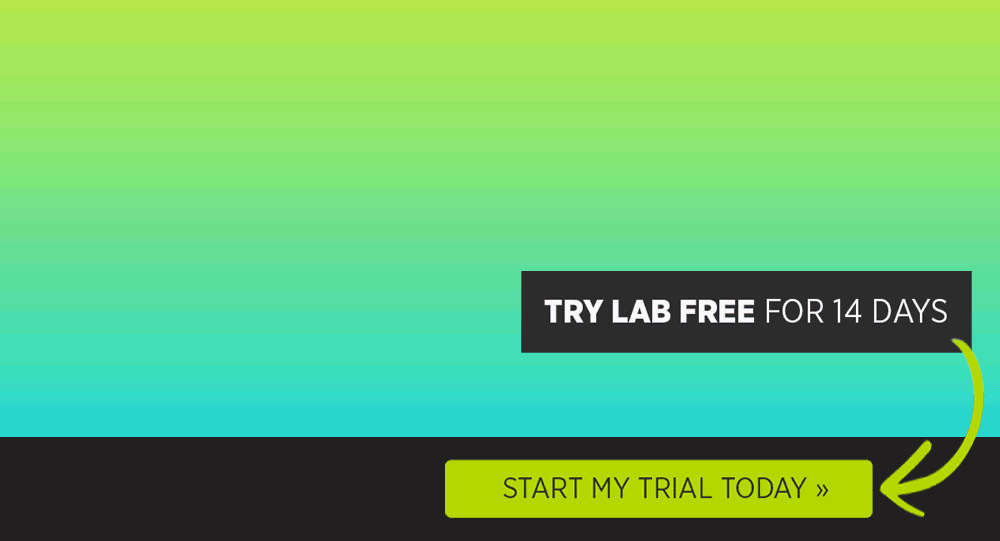
The post Digital Marketing Data and How to Optimize Like a Champ appeared first on DigitalMarketer.